Table of Contents
Introduction
NLP is a branch of data science that consists of systematic processes for analyzing, understanding, and deriving information from the text data in a smart and efficient manner.
Natural Language Processing can be stated in layman terms as the automatic processing of the natural human language by a machine. It is a specialized branch of Artificial Intelligence that primarily focuses on interpretation as well as human-generated data – text or speech-based.
Perform numerous automated tasks and solve a wide range of problems such as – automatic summarization, machine translation, named entity recognition, relationship extraction, sentiment analysis, speech recognition, and topic segmentation, etc. The automatic manipulation of natural languages, like speech and text, by software.
NLP is a field of Artificial intelligence that gives the machines the ability to read, understand and derive meaning from human Languages.
But there is a problem: one person may generate hundreds or thousands of words in a declaration, each sentence with its corresponding complex or twist in a sentence. If you want to analyze this hundred, thousands, or millions of people or declarations in a given geography, then the situation is unmanageable. Thanks to the advances in disciplines like machine learning, a big revolution is going on regarding this topic.
Widget not in any sidebars
Use Cases of NLP
- Organizations can determine what customers are saying about a service or review of the product by identifying and extracting information in sources like social media. This sentiment analysis can provide a lot of information about customer’s choices and their decision drivers.
- Email Classification i.e. spam or not spam. Companies like Yahoo and Google filter and classify your emails with NLP by analyzing text in emails that flow through their servers and stopping spam before they even enter your inbox.
- Amazon’s Alexa and Apple’s Siri and Google Assitance are examples of intelligent voice-driven interfaces that use NLP to respond to vocal prompts and do everything like find a particular shop, tell us the weather forecast, suggest the best song, or turn on the lights at home.
NLP is particularly booming in the healthcare industry. This technology is improving detect the disease, detect the damaged part of our body, and bringing costs down while healthcare organizations are going through a growing adoption of electronic health records.
Natural Language Processing in Healthcare has two use cases.
- Comprehending human speech and extracting its meaning
- Uncloaking unstructured data in the database and documents by mapping out essential concepts as well as values and allowing physicians to use this information for decision making and analytics.
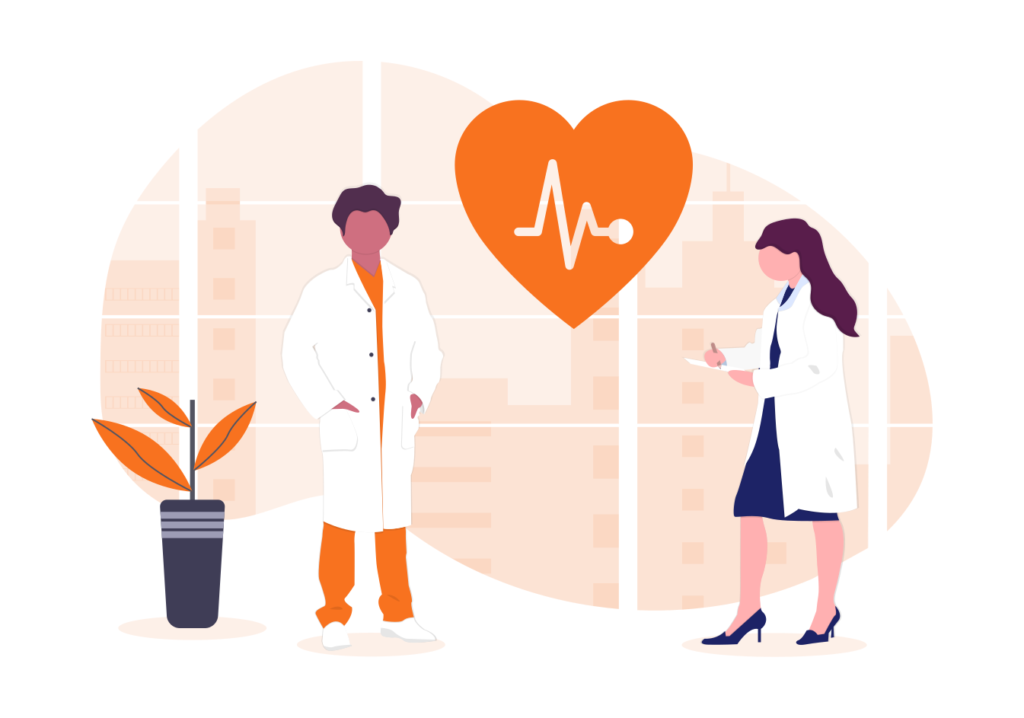
- Improvement in Clinical Documentation – Machine Learning in healthcare has touched clinical documentation, freeing up physicians from the manual and complex structure of EHRs, allowing them to focus more on care delivery. This has been possible because of speech-to-text. As machine learning in healthcare advances, we will be able to pull pertinent data from other emerging sources.
Implementing Predictive Analytics in Healthcare
Identification of high-risk patients, as well as improvement of the diagnosis process, can be done by deploying Predictive Analytics along with Natural Language Processing in Healthcare along with predictive analytics. An NLP based algorithm identified at-risk patients of Kawasaki disease with a sensitivity of 93.6% and specificity of 77.5% compared to the manual review of clinician’s notes.
NLP helped in rendering unstructured data which was then used to identify early signs and intimate clinicians accordingly.
This recalls the case of Google Flu Trends which in 2009 was announced as being able to predict influenza but later on vanished due to its low accuracy and inability to meet its projected rates.
Future Work
At the moment NLP is battling to detect nuances in language meaning, whether due to lack of context, spelling errors, or speech error while taking, translate mistake, and many more.
In March 2016 Microsoft launched Tay, an Artificial Intelligence (AI) chatbot released on Twitter as an NLP experiment. The idea was that as more users conversed with Tay, the smarter it would get. Well, the result was that after 16 hours Tay had to be removed due to its racist and abusive comments:
Microsoft learned from its own experience and some months later released Zo, its second-generation English-language chatbot that won’t be caught making the same mistakes as its predecessor. Zo uses a combination of innovative approaches to recognize and generate conversation, and other companies are exploring with bots that can remember details specific to an individual conversation.
Although the future looks extremely challenging and full of threats for NLP, the discipline is developing at a very fast pace (probably like never before) and we are likely to reach a level of advancement in the coming years that will make complex applications look possible.
Conclusion
In this article, we are learning overview of Natural Language Processing(NLP).With an application where it is used.