Table of Contents
How We Can Use Data Science In Banking?
The Application of Data Science in Banking would have made you realize that data science cannot let any aspect of modern-day human civilization remain untouched including the healthcare industry also. We all, in some manner, are living under the consequence of its “Midas touch”.
Time now to talk about another beneficiary – the eternal love of human life – MONEY. Before you race your wild horses, let me tell you that this Midas touch is not turning your money into gold. But, it is proving golden for the incubators of your money – the Banks.
Yes, being the industry it is, banking has fast adapted to technology to make its operational procedures more efficient, secure and quick.
The insights, results, and customer retention have been nothing short of magnificent. Data Science has delivered wonderfully for the banks– it had to, for, a lot many bucks were at stake.
Take a while, or maybe more to ponder over these “wonders”:
Risk Modeling
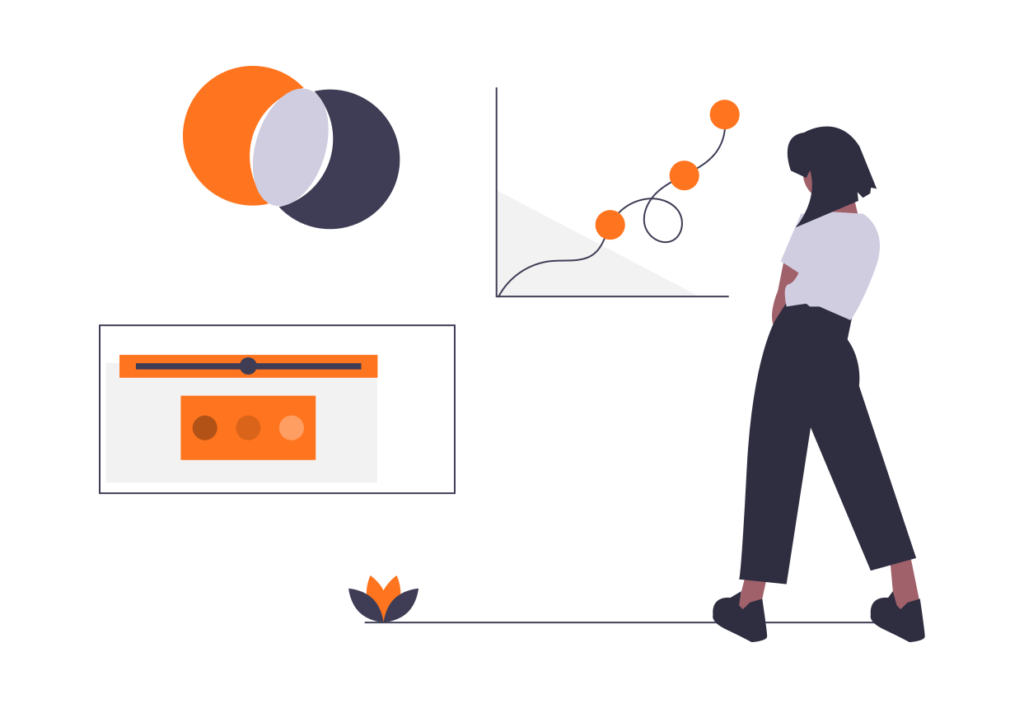
Call it a “Health Check” for a bank’s financial condition. Data science in banking is used for Risk modeling assesses a bank’s performance and helps it formulate plans for the future. It softens a certain risk and helps banks tackle it better by making effective data-driven decisions.
Banks require risk modeling when they look to evaluate a company’s worth for large monetary settlements, corporate funding, partnerships/acquisitions, infrastructural or organizational shuffling, etc.
Certainly with good backing from valuable data and smart insights, decision making in such cases becomes easier for banks.
Customer Segmentation

Another example of data science in banking is Customer Segmentation is an integral process for banking operations. It helps the banks identify groups of customers based on their behavioral instincts, buying patterns, and demographic features.
Segmentation of customers gives the banks a better idea of targeting ideal customers suited for a particular scheme. It also determines the resources to be invested in each segment of the customer.
Data Science has always been a champion at this cause – it has loads of techniques for classification. It perfectly uses clustering and classification to chalk out value-based segments of customers for the banks to appropriately target their approach, attention, and plans. K-means, Logistic Regression, Decision Trees, etc. become the best friends of banks (read Data scientists with banking clients) for these purposes.
Widget not in any sidebars
Find me a better example of “friends with benefits”. Will ya?!!
Real-time Predictive Analytics

Mandatory component alert!
Only a fool will be wise enough to let go of Predictive Analytics for ANY business. And we all know how smart our banks are!
With a solar system full of data available at their disposal, banks need to identify patterns, derive analytics and predict consequences using machine learning algorithms and data science techniques.
Once a machine learning algorithm predicts the performance of a component, banks can then design strategies to upscale growth, counter meltdowns, aggravate approach, and retain customers. It not only helps in enhancing a well-performing unit but also prevents a potential future downfall.
Fraud Detection
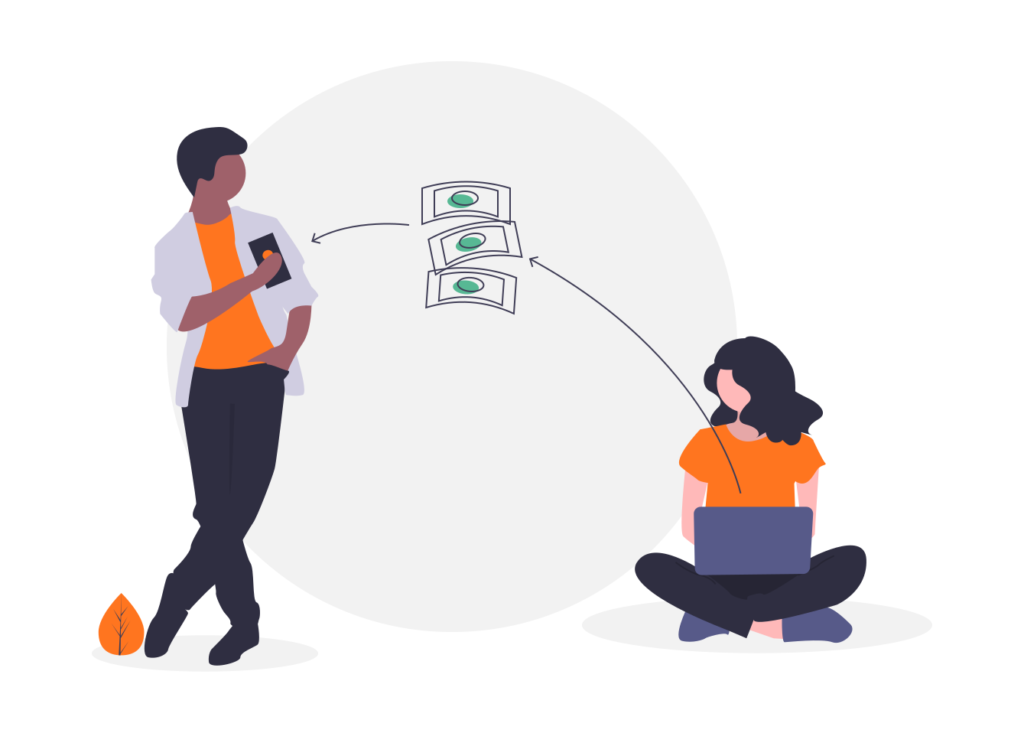
This is mostly used application of data science in banking for fraud detection in finance and fraud go hand-in-hand! You certainly cannot deny the truth quotient of this statement.
Banks have to be cautious and extremely vigilant of all transactions taking place. And with the ever-increasing online behaviour, attackers and hackers are ever ready to pounce upon.
Data Science lends a hand by identifying unusual transactional patterns and customer behavioral anomalies. Any departure from normal is given serious attention – ML algorithms K-means clustering and Support Vector Machines are increasingly employed for the purpose.
Lifetime Value Prediction

Banks have a primary target of building long term relationships with their customers. Like all good relationships in the world, this too requires due consideration and attention.
This process is about determining the overall value that a customer adds during his/her association with the bank.
Data Science in banking serves the cause by analyzing the value derived from each of the customer’s interactions with the bank and also predicts probable future actions. It helps the banks in serving the demands of customers and also aids in planning customer retention strategies.
The goal is also to nurture, maintain and sustain the relationship for which a rigorous methodology is to be followed. Lifetime Value requires extensive cleaning, manipulation, profiling, and segmentation to generate models that “fit” to both – the banks and its customers.
Collaborative Filtering

Who doesn’t enjoy receiving personalized recommendations here? Be it the shows and movies that Netflix suggests us or the interest-based Google app stories – all of us are fond of these tailor-made experiences.
Can this be replicated in our financial behavior with the use of data science in banking? Of course, why not says Data Science – an eternal optimistic and challenge-driven creature.
It uses tools and algorithms to suggest the most suitable items for a customer. The idea is to find a correlation in the consumption patterns of the customer. This is based on historic interaction, activity, demographic consideration and buying behaviour.
The process is driven by keeping the preferences of each customer in mind which improves the overall buying experience. It also saves a customer the time of searching for an offer that best suits his/her needs – banks readily present (read serve) the same.
Customer Support

Talk of interaction and who can forget the never-ending calls for loans, investments, and insurance plans?
Well, the intervention of Data Science in Banking makes things better! How?
It identifies your interaction with banking schemes, favourable investment scenarios, and monetary records. It then asks the customer support to call you only for the loans you direly need or the investment that you are highly interested in. This results in longer, better, more relevant and customer-centric conversations.
What also comes under the same category is 24/7 availability through chatbots, online query solutions, tracking of problems, and rapid complaint redressals. So now you won’t avoid those calls – right?? But, there are lots involved to make the scenario pleasing – bots, automation, rigorous analysis, feature selection, and whatnot.
And now I see you are smiling like a happy customer – so are the banks. Thank Data Science later.
Look around yourself and you will find these applications being implemented effectively – the deadly combination of the boon and the buzzword has been “cashed-in” perfectly.
Give it a thought – it won’t cost you much. Given “effective” is taken seriously, the banks won’t see any other defaulter flying away (literally) with their 9000 crores, leaving behind only a defunct airline and wasted liquor to look at.
I hope the thought didn’t cost what you just read.
Widget not in any sidebars
Jokes apart, this is how Data Science finds its place in the industrious, stressful, chaotic and monetarily fueled life of banks. Data Science aims to make it less of a stress and looks to squash the chaos.
It aims to make banking a data-driven, technologically inspired, and relationship committed business (“monetarily-fueled” has to, of course, remain).